Compute configuration reference
This article explains the configuration settings available in the Create Compute UI. Most users create compute resources using their assigned policies, which limits the configurable settings. If you don’t see a particular setting in your UI, it’s because the policy you’ve selected does not allow you to configure that setting.
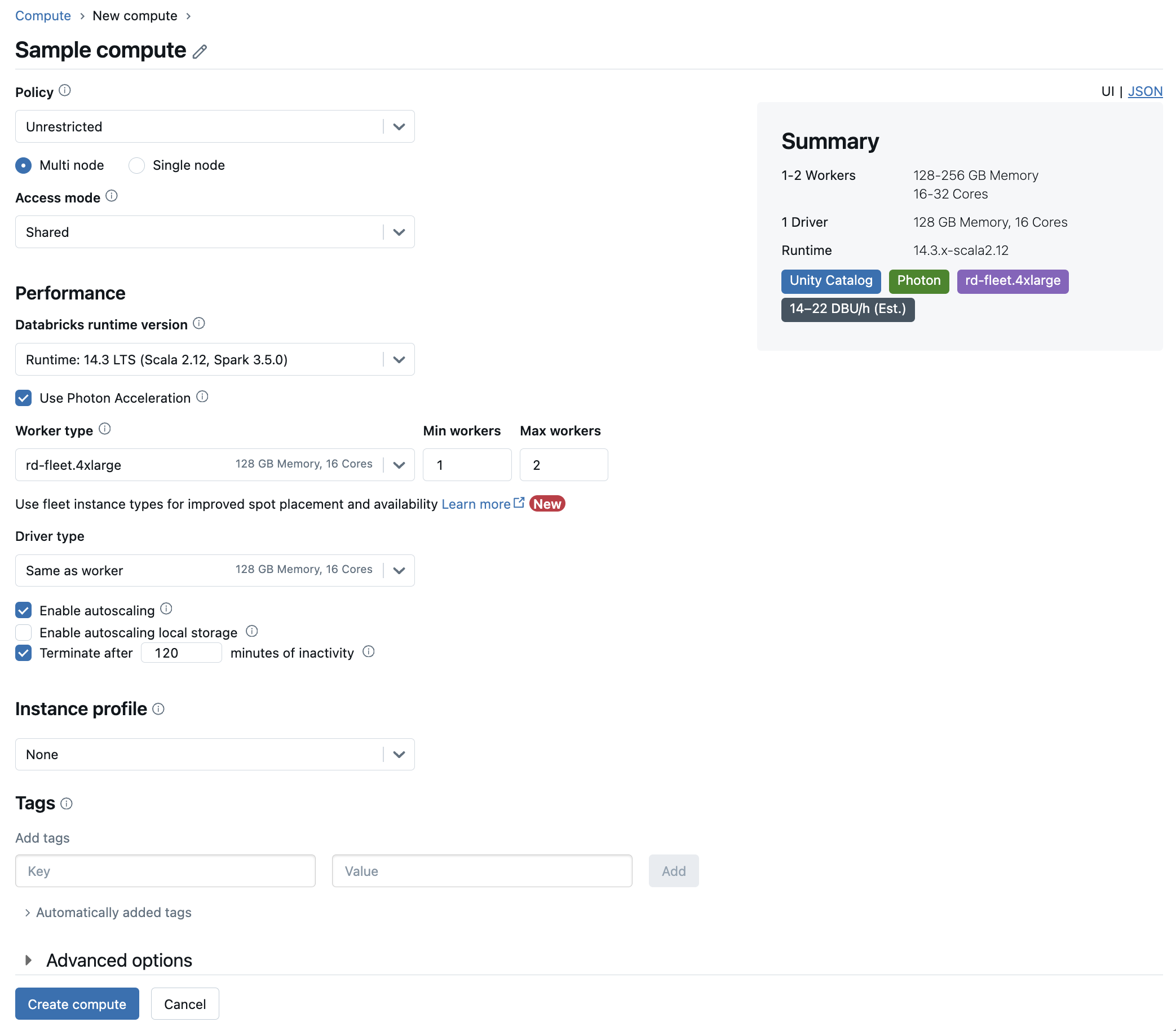
The configurations and management tools described in this article apply to both all-purpose and job compute. For more considerations on configuring job compute, see Configure compute for jobs.
Create a new all-purpose compute resource
To create a new all-purpose compute resource:
In the workspace sidebar, click Compute.
Click the Create compute button.
Configure the compute resource.
Click Create compute.
You new compute resource will automatically start spinning up and be ready to use shortly.
Policies
Policies are a set of rules used to limit the configuration options available to users when they create compute resources. If a user doesn’t have the Unrestricted cluster creation entitlement, then they can only create compute resources using their granted policies.
To create compute resources according to a policy, select a policy from the Policy drop-down menu.
By default, all users have access to the Personal Compute policy, allowing them to create single-machine compute resources. If you need access to Personal Compute or any additional policies, reach out to your workspace admin.
Single-node or multi-node compute
Depending on the policy, you can select between creating a Single node compute resource or a Multi node compute resource.
Single node compute is intended for jobs that use small amounts of data or non-distributed workloads such as single-node machine learning libraries. Multi-node compute should be used for larger jobs with distributed workloads.
Single node properties
A single node compute resource has the following properties:
Runs Spark locally.
Driver acts as both master and worker, with no worker nodes.
Spawns one executor thread per logical core in the compute resource, minus 1 core for the driver.
Saves all
stderr
,stdout
, andlog4j
log outputs in the driver log.Can’t be converted to a multi-node compute resource.
Selecting single or multi node
Consider your use case when deciding between single or multi-node compute:
Large-scale data processing will exhaust the resources on a single node compute resource. For these workloads, Databricks recommends using multi-node compute.
Single-node compute is not designed to be shared. To avoid resource conflicts, Databricks recommends using a multi-node compute resource when the compute must be shared.
A multi-node compute resource can’t be scaled to 0 workers. Use single node compute instead.
Single-node compute is not compatible with process isolation.
GPU scheduling is not enabled on single node compute.
On single-node compute, Spark cannot read Parquet files with a UDT column. The following error message results:
The Spark driver has stopped unexpectedly and is restarting. Your notebook will be automatically reattached.
To work around this problem, disable the native Parquet reader:
spark.conf.set("spark.databricks.io.parquet.nativeReader.enabled", False)
Access modes
Access mode is a security feature that determines who can use the compute resource and the data they can access using the compute resource. Every compute resource in Databricks has an access mode.
Databricks recommends that you use shared access mode for all workloads. Use the single user access mode only if your required functionality is not supported by shared access mode.
Access Mode |
Visible to user |
UC Support |
Supported Languages |
Notes |
---|---|---|---|---|
Single user |
Always |
Yes |
Python, SQL, Scala, R |
Can be assigned to and used by a single user. Referred to as Assigned access mode in some workspaces. |
Shared |
Always (Premium plan required) |
Yes |
Python (on Databricks Runtime 11.3 LTS and above), SQL, Scala (on Unity Catalog-enabled compute using Databricks Runtime 13.3 LTS and above) |
Can be used by multiple users with data isolation among users. |
No Isolation Shared |
Admins can hide this access mode by enforcing user isolation in the admin settings page. |
No |
Python, SQL, Scala, R |
There is a related account-level setting for No Isolation Shared compute. |
Custom |
Hidden (For all new compute) |
No |
Python, SQL, Scala, R |
This option is shown only if you have an existing compute resource without a specified access mode. |
You can upgrade an existing compute resource to meet the requirements of Unity Catalog by setting its access mode to Single User or Shared. For detailed information about the functionality that is supported by each of these access modes in Unity Catalog-enabled workspaces, see Compute access mode limitations for Unity Catalog.
Note
In Databricks Runtime 13.3 LTS and above, init scripts and libraries are supported by all access modes. Requirements and levels of support vary. See Where can init scripts be installed? and Cluster-scoped libraries.
Databricks Runtime versions
Databricks Runtime is the set of core components that run on your compute. Select the runtime using the Databricks Runtime Version drop-down menu. For details on specific Databricks Runtime versions, see Databricks Runtime release notes versions and compatibility. All versions include Apache Spark. Databricks recommends the following:
For all-purpose compute, use the most current version to ensure you have the latest optimizations and the most up-to-date compatibility between your code and preloaded packages.
For job compute running operational workloads, consider using the Long Term Support (LTS) Databricks Runtime version. Using the LTS version will ensure you don’t run into compatibility issues and can thoroughly test your workload before upgrading.
For data science and machine learning use cases, consider Databricks Runtime ML version.
Use Photon acceleration
Photon is available on compute running Databricks Runtime 9.1 LTS and above.
To enable or disable Photon acceleration, select the Use Photon Acceleration checkbox. To learn more about Photon, see What is Photon?.
Worker and driver node types
A compute resource consists of one driver node and zero or more worker nodes. You can pick separate cloud provider instance types for the driver and worker nodes, although by default the driver node uses the same instance type as the worker node. Different families of instance types fit different use cases, such as memory-intensive or compute-intensive workloads.
You can also select a pool to use as the worker or driver node. Only use a pool with preemptible VM instances as your worker type. Select a separate on-demand driver type to prevent your driver from being reclaimed. See Connect to pools.
Worker type
In multi-node compute, worker nodes run the Spark executors and other services required for a properly functioning compute resource. When you distribute your workload with Spark, all of the distributed processing happens on worker nodes. Databricks runs one executor per worker node. Therefore, the terms executor and worker are used interchangeably in the context of the Databricks architecture.
Tip
To run a Spark job, you need at least one worker node. If the compute resource has zero workers, you can run non-Spark commands on the driver node, but Spark commands will fail.
Worker node IP addresses
Databricks launches worker nodes with two private IP addresses each. The node’s primary private IP address hosts Databricks internal traffic. The secondary private IP address is used by the Spark container for intra-cluster communication. This model allows Databricks to provide isolation between multiple compute resources in the same workspace.
Driver type
The driver node maintains state information of all notebooks attached to the compute resource. The driver node also maintains the SparkContext, interprets all the commands you run from a notebook or a library on the compute resource, and runs the Apache Spark master that coordinates with the Spark executors.
The default value of the driver node type is the same as the worker node type. You can choose a larger driver node type with more memory if you are planning to collect()
a lot of data from Spark workers and analyze them in the notebook.
Tip
Since the driver node maintains all of the state information of the notebooks attached, make sure to detach unused notebooks from the driver node.
GPU instance types
For computationally challenging tasks that demand high performance, like those associated with deep learning, Databricks supports compute resources that are accelerated with graphics processing units (GPUs). For more information, see GPU-enabled compute.
Preemptible instances
A preemptible VM instance is an instance that you can create and run at a much lower price than normal instances. However, Google Cloud might stop (preempt) these instances if it requires access to those resources for other tasks. Preemptible instances use excess Google Compute Engine capacity, so their availability varies with usage.
When you create a new compute resource, you can enable preemptible VM instances in two different ways:
When you create compute using the UI, click preemptible instances next to the Worker Type details.
When you create an instance pool using the UI, set On-Demand/Preemptible to All preemptible, Preemptible with fallback GCP, or On demand GCP. If preemptible VM instances are not available, by default the compute will fall back to using on-demand VM instances. To configure the fall-back behavior, set
gcp_attributes.gcp_availability
toPREEMPTIBLE_GCP
orPREEMPTIBLE_WITH_FALLBACK_GCP
. The default isON_DEMAND_GCP
.
{
"instance_pool_name": "Preemptible w/o fallback API test",
"node_type_id": "n1-highmem-4",
"gcp_attributes": {
"availability": "PREEMPTIBLE_GCP"
}
}
Next, create a new compute resource and set Pool to a preemptible instance pool.
Instance types with local SSDs
For the latest list of instance types, the prices of each, and the size of the local SSDs, see the GCP pricing estimator.
Instance types that have local SSDs are encrypted with default Google Cloud server-side encryption and automatically use disk caching for improved performance. Cache sizes on all instance types are set automatically, so you do not need to set the disk usage explicitly.
Configure local SSDs for your compute
You can configure the number of local SSDs to attach to your compute resource when you use the Clusters API to create your compute resource.
To configure the number of local SSDs, set a value for local_ssd_count
in the gcp_attributes
object. Each instance type can only support a certain number of attached local SSDs. The value specified in local_ssd_count
must be valid for both the driver and worker instance type. For more information, see the GCP doc for Local SSDs and machine types.
Enable autoscaling
When Enable autoscaling is checked, you can provide a minimum and maximum number of workers for the compute resource. Databricks then chooses the appropriate number of workers required to run your job.
To set the minimum and the maximum number of workers your compute resource will autoscale between, use the Min workers and Max workers fields next to the Worker type dropdown.
If you don’t enable autoscaling, you must enter a fixed number of workers in the Workers field next to the Worker type dropdown.
Note
When the compute resource is running, the compute details page displays the number of allocated workers. You can compare number of allocated workers with the worker configuration and make adjustments as needed.
Benefits of autoscaling
With autoscaling, Databricks dynamically reallocates workers to account for the characteristics of your job. Certain parts of your pipeline may be more computationally demanding than others, and Databricks automatically adds additional workers during these phases of your job (and removes them when they’re no longer needed).
Autoscaling makes it easier to achieve high utilization because you don’t need to provision the compute to match a workload. This applies especially to workloads whose requirements change over time (like exploring a dataset during the course of a day), but it can also apply to a one-time shorter workload whose provisioning requirements are unknown. Autoscaling thus offers two advantages:
Workloads can run faster compared to a constant-sized under-provisioned compute resource.
Autoscaling can reduce overall costs compared to a statically-sized compute resource.
Depending on the constant size of the compute resource and the workload, autoscaling gives you one or both of these benefits at the same time. The compute size can go below the minimum number of workers selected when the cloud provider terminates instances. In this case, Databricks continuously retries to re-provision instances in order to maintain the minimum number of workers.
Note
Autoscaling is not available for spark-submit
jobs.
Note
Compute auto-scaling has limitations scaling down cluster size for Structured Streaming workloads. Databricks recommends using Delta Live Tables with enhanced autoscaling for streaming workloads. See Optimize the cluster utilization of Delta Live Tables pipelines with enhanced autoscaling.
How autoscaling behaves
Autoscaling has the following characteristics:
Starts by adding 8 nodes. Then scales up exponentially, taking as many steps as required to reach the max.
Scales down when 90% of the nodes are not busy for 10 minutes and the compute has been idle for at least 30 seconds.
Scales down exponentially, starting with 1 node.
Autoscaling with pools
If you are attaching your compute resource to a pool, consider the following:
Make sure the compute size requested is less than or equal to the minimum number of idle instances in the pool. If it is larger, compute startup time will be equivalent to compute that doesn’t use a pool.
Autoscaling example
If you reconfigure a static compute resource to autoscale, Databricks immediately resizes the compute resource within the minimum and maximum bounds and then starts autoscaling. As an example, the following table demonstrates what happens to a compute resource with a certain initial size if you reconfigure the compute resource to autoscale between 5 and 10 nodes.
Initial size |
Size after reconfiguration |
---|---|
6 |
6 |
12 |
10 |
3 |
5 |
Enable autoscaling local storage
Google Cloud compute instances can be supplemented with additional storage at the worker level using zonal solid state persistent disks.
With autoscaling local storage, Databricks monitors the amount of free disk space available on your compute’s Spark workers. If a worker begins to run too low on disk, Databricks automatically resizes the zonal SSD PD before it runs out of disk space. Zonal-SSD PD volumes are attached up to a limit of 5 TB of total disk space per instance (including the instance’s local storage).
To configure autoscaling storage, select Enable autoscaling local storage.
Default provisioned storage
Databricks provisions the following storage for each worker node:
One boot disk per instance that is used by the host operating system and Databricks internal services. The boot disk is 100 GB for non-GPU instances and 200 GB for GPU instances.
Local SSD used by the Spark worker. This hosts Spark services and logs. Each local SSD is 375GB. To configure the number of attached local SSDs, see Instance types with local SSDs.
Remote SSDs when storage autoscaling is enabled. These start at 150 GB at creation and autoscale as needed.
Local disk encryption
Instance types that have local SSDs are encrypted with default Google Cloud server-side encryption. See Instance types with local SSDs.
Automatic termination
You can set auto termination for compute. During compute creation, specify an inactivity period in minutes after which you want the compute resource to terminate.
If the difference between the current time and the last command run on the compute resource is more than the inactivity period specified, Databricks automatically terminates that compute. resource For more information on compute termination, see Terminate a compute.
Tags
Tags allow you to easily monitor the cost of cloud resources used by various groups in your organization. Specify tags as key-value pairs when you create compute, and Databricks applies these tags to Databricks Runtime pods and persistent volumes on the GKE cluster and to DBU usage reports.
The Databricks billable usage graphs in the account console can aggregate usage by individual tags. The billable usage CSV reports downloaded from the same page also include default and custom tags. Tags also propagate to GKE and GCE labels.
For detailed information about how pool and compute tag types work together, see Monitor usage using tags
To add tags to your compute resource:
In the Tags section, add a key-value pair for each custom tag.
Click Add.
Google service account
To associate this compute resource with a Google service account using Google Identity, click Advanced Options and add your Google service account email address in the Google Service Account field. This value is used to authenticate with the GCS and BigQuery data sources.
Important
The service account that you use to access GCS and BigQuery data sources must be in the same project as the service account that was specified when setting up your Databricks account.
Availability zones
In the compute configuration page, under Advanced options, select the compute resource’s availability zone. This setting lets you specify which availability zone you want the compute resource to use. By default, the availability zone setting is set to Auto. With a setting of Auto, a single availability zone is automatically picked for you.
You can also choose a specific zone. Choosing a specific zone is useful primarily if your organization has purchased reserved instances in specific availability zones.
High availability zone
You can also select HA
as the availability zone. High availability (HA) is a system feature designed to provide a consistent level of uptime for prolonged periods. Using an HA
zone configuration can reduce the probability of single-zone availability issues, such as zonal unavailability or being unable to source instance capacity in a zone.
When HA
is selected as the availability zone, Databricks balances instance placement across the zones in a region. This could lead to an increase in price through inter-zone egress charges.
Spark configuration
To fine-tune Spark jobs, you can provide custom Spark configuration properties.
On the compute configuration page, click the Advanced Options toggle.
Click the Spark tab.
In Spark config, enter the configuration properties as one key-value pair per line.
When you configure compute using the Clusters API, set Spark properties in the spark_conf
field in the create cluster API or Update cluster API.
To enforce Spark configurations on compute, workspace admins can use compute policies.
Retrieve a Spark configuration property from a secret
Databricks recommends storing sensitive information, such as passwords, in a secret instead of plaintext. To reference a secret in the Spark configuration, use the following syntax:
spark.<property-name> {{secrets/<scope-name>/<secret-name>}}
For example, to set a Spark configuration property called password
to the value of the secret stored in secrets/acme_app/password
:
spark.password {{secrets/acme-app/password}}
For more information, see Manage secrets.
Environment variables
Configure custom environment variables that you can access from init scripts running on the compute resource. Databricks also provides predefined environment variables that you can use in init scripts. You cannot override these predefined environment variables.
On the compute configuration page, click the Advanced Options toggle.
Click the Spark tab.
Set the environment variables in the Environment Variables field.
Note
ENV
is a reserved word and cannot be used as the name of an environment variable.
You can also set environment variables using the spark_env_vars
field in the Create cluster API or Update cluster API.
Compute log delivery
When you create compute, you can specify a location to deliver the logs for the Spark driver node, worker nodes, and events. Logs are delivered every five minutes and archived hourly in your chosen destination. When a compute resource is terminated, Databricks guarantees to deliver all logs generated up until the compute resource was terminated.
The destination of the logs depends on the compute resource’s cluster_id
. If the specified destination is
dbfs:/cluster-log-delivery
, compute logs for 0630-191345-leap375
are delivered to
dbfs:/cluster-log-delivery/0630-191345-leap375
.
To configure the log delivery location:
On the compute page, click the Advanced Options toggle.
Click the Logging tab.
Select a destination type.
Enter the compute log path.
The log path must be a DBFS path that begins with
dbfs:/
.
Note
This feature is also available in the REST API. See the Clusters API.